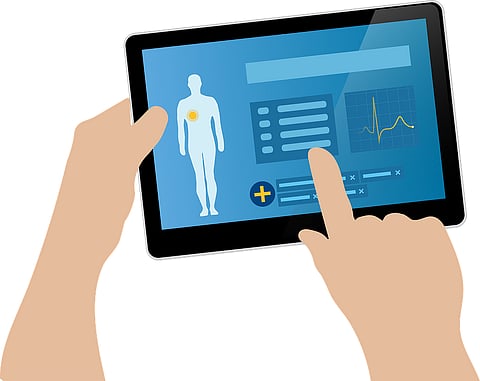
Electronic medical records (EMRs) are digital versions of the paper charts typically found in clinician offices, clinics, and hospitals and are mostly used by healthcare providers for diagnosis and treatment. New research has leveraged EMR data analysis to predict the risk of developing adverse outcomes in the near future based on an individual’s medical history, and also pinpoint which medications may have beneficial effects. These results will be shared at the 47th annual scientific meeting of the Research Society on Alcohol (RSA) in Minneapolis, Minnesota.
“EMRs contain a lot of information,” said Lirong Wang, assistant professor of pharmaceutical sciences at the University of Pittsburgh, “including diagnosis, medication use, lab tests, procedures, medical notes by physicians, and even images such as computerized tomography (CT) scans and magnetic resonance (MR) imaging. The data contained within EMRs can offer valuable insights into disease trajectory and progression, co-morbidities, and various treatments options. A deep-learning model is a kind of predictive tool that mimics the complex decision-making power of the human brain by analyzing data to identify and classify phenomena, recognize patterns and relationships, and evaluate possibilities.”
Wang will discuss her team’s research at the RSA meeting on 23 June 2024.
Researchers analyzed the EMRs of 5,565 high-risk patients with co-occurring post-traumatic stress disorder (PTSD) and alcohol use disorder (AUD) from the University of Pittsburgh Medical Center. They developed and applied a deep learning model to predict adverse events such as opioid use disorder, suicide related events, depression, and death within three months after the PTSD/AUD diagnosis. They also analyzed input data such as diagnosis, medication, lab tests, co-morbidities, and individual- and neighborhood-level social determinants of health (SDoH) to find which medications and therapies could improve outcomes.
“We made three key findings,” said Wang. “One, we can predict patient outcomes by applying deep learning models to electronic medical records. Two, we can identify risk factors such as individual and neighborhood level SDoH that are associated with adverse outcomes. Three, we can suggest medications that can help avoid adverse outcomes. In summary, we have shown that by leveraging the large volume of EMRs in conjunction with deep learning approaches, we can gather real-world evidence to support optimal treatment of patients with PTSD and AUD.”