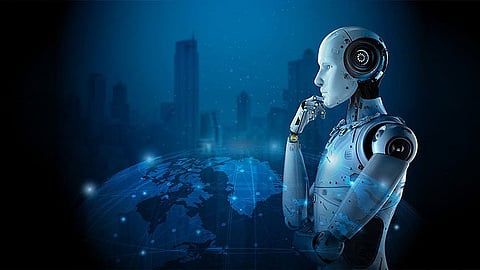
An interdisciplinary team of researchers from the University of Jyväskylä, Finland, from the fields of Psychology, Education, and Information Technology, documented pioneering findings. They developed the first machine learning models that forecasted upper secondary education dropout, earlier than ever before. By utilizing a 13-year longitudinal dataset with onset in kindergarten age, the models predicted secondary school education dropout and retention from as early as the end of primary school (Grade 6).
"This study marks a significant advancement in early automatic classification, but it is just the first step in a methodological development to be continued. Such an approach could set a new precedent for enhancing existing student retention and success strategies, potentially leading to transformative changes in educational systems and policies," says Maria Psyridou, post-doctoral researcher and lead author of the study.
Harnessing Early Data
The process of dropping out of school often begins in the early school years and is influenced by a range of different factors. This study utilized 13 years of longitudinal data from the “First Steps” study and its extension, the “School Path” focusing on Secondary and Higher Education, both funded by the Research Council of Finland. The data encompass both family background, and individual factors, behavioural measures, motivation and engagement metrics, health behaviours and experiences of bullying, media usage, and academic and cognitive performance.
"Working with this longitudinal data presented both a challenge and a unique opportunity for machine learning. The results are really promising," adds Fabi Prezja, the doctoral researcher who co-developed the machine learning approach for this study.
Planning for the Future
The study represents a significant leap forward in educational research. However, additional data, and further validation using independent test sets are essential. In future iterations, such models may have the potential to proactively support educational processes and existing protocols for identifying at-risk students, thereby potentially aiding in the reinvention of student retention and success strategies, and ultimately contributing to improved educational outcomes.